#HTE
Presenting onstage today in the 2018 TC Disrupt Berlin Battlefield is Indian agtech startup Imago AI, which is applying AI to help feed the world’s growing population by increasing crop yields and reducing food waste. As startup missions go, it’s an impressively ambitious one.
The team, which is based out of Gurgaon near New Delhi, is using computer vision and machine learning technology to fully automate the laborious task of measuring crop output and quality — speeding up what can be a very manual and time-consuming process to quantify plant traits, often involving tools like calipers and weighing scales, toward the goal of developing higher-yielding, more disease-resistant crop varieties.
Currently they say it can take seed companies between six and eight years to develop a new seed variety. So anything that increases efficiency stands to be a major boon.
And they claim their technology can reduce the time it takes to measure crop traits by up to 75 percent.
In the case of one pilot, they say a client had previously been taking two days to manually measure the grades of their crops using traditional methods like scales. “Now using this image-based AI system they’re able to do it in just 30 to 40 minutes,” says co-founder Abhishek Goyal.
Using AI-based image processing technology, they can also crucially capture more data points than the human eye can (or easily can), because their algorithms can measure and asses finer-grained phenotypic differences than a person might pick up on or be easily able to quantify just judging by eye alone.
“Some of the phenotypic traits they are not possible to identify manually,” says co-founder Shweta Gupta. “Maybe very tedious or for whatever all these laborious reasons. So now with this AI-enabled [process] we are now able to capture more phenotypic traits.
“So more coverage of phenotypic traits… and with this more coverage we are having more scope to select the next cycle of this seed. So this further improves the seed quality in the longer run.”
The wordy phrase they use to describe what their technology delivers is: “High throughput precision phenotyping.”
Or, put another way, they’re using AI to data-mine the quality parameters of crops.
“These quality parameters are very critical to these seed companies,” says Gupta. “Plant breeding is a very costly and very complex process… in terms of human resource and time these seed companies need to deploy.
“The research [on the kind of rice you are eating now] has been done in the previous seven to eight years. It’s a complete cycle… chain of continuous development to finally come up with a variety which is appropriate to launch in the market.”
But there’s more. The overarching vision is not only that AI will help seed companies make key decisions to select for higher-quality seed that can deliver higher-yielding crops, while also speeding up that (slow) process. Ultimately their hope is that the data generated by applying AI to automate phenotypic measurements of crops will also be able to yield highly valuable predictive insights.
Here, if they can establish a correlation between geotagged phenotypic measurements and the plants’ genotypic data (data which the seed giants they’re targeting would already hold), the AI-enabled data-capture method could also steer farmers toward the best crop variety to use in a particular location and climate condition — purely based on insights triangulated and unlocked from the data they’re capturing.
One current approach in agriculture to selecting the best crop for a particular location/environment can involve using genetic engineering. Though the technology has attracted major controversy when applied to foodstuffs.
Imago AI hopes to arrive at a similar outcome via an entirely different technology route, based on data and seed selection. And, well, AI’s uniform eye informing key agriculture decisions.
“Once we are able to establish this sort of relation this is very helpful for these companies and this can further reduce their total seed production time from six to eight years to very less number of years,” says Goyal. “So this sort of correlation we are trying to establish. But for that initially we need to complete very accurate phenotypic data.”
“Once we have enough data we will establish the correlation between phenotypic data and genotypic data and what will happen after establishing this correlation we’ll be able to predict for these companies that, with your genomics data, and with the environmental conditions, and we’ll predict phenotypic data for you,” adds Gupta.
“That will be highly, highly valuable to them because this will help them in reducing their time resources in terms of this breeding and phenotyping process.”
“Maybe then they won’t really have to actually do a field trial,” suggests Goyal. “For some of the traits they don’t really need to do a field trial and then check what is going to be that particular trait if we are able to predict with a very high accuracy if this is the genomics and this is the environment, then this is going to be the phenotype.”
So — in plainer language — the technology could suggest the best seed variety for a particular place and climate, based on a finer-grained understanding of the underlying traits.
In the case of disease-resistant plant strains it could potentially even help reduce the amount of pesticides farmers use, say, if the the selected crops are naturally more resilient to disease.
While, on the seed generation front, Gupta suggests their approach could shrink the production time frame — from up to eight years to “maybe three or four.”
“That’s the amount of time-saving we are talking about,” she adds, emphasizing the really big promise of AI-enabled phenotyping is a higher amount of food production in significantly less time.
As well as measuring crop traits, they’re also using computer vision and machine learning algorithms to identify crop diseases and measure with greater precision how extensively a particular plant has been affected.
This is another key data point if your goal is to help select for phenotypic traits associated with better natural resistance to disease, with the founders noting that around 40 percent of the world’s crop load is lost (and so wasted) as a result of disease.
And, again, measuring how diseased a plant is can be a judgement call for the human eye — resulting in data of varying accuracy. So by automating disease capture using AI-based image analysis the recorded data becomes more uniformly consistent, thereby allowing for better quality benchmarking to feed into seed selection decisions, boosting the entire hybrid production cycle.
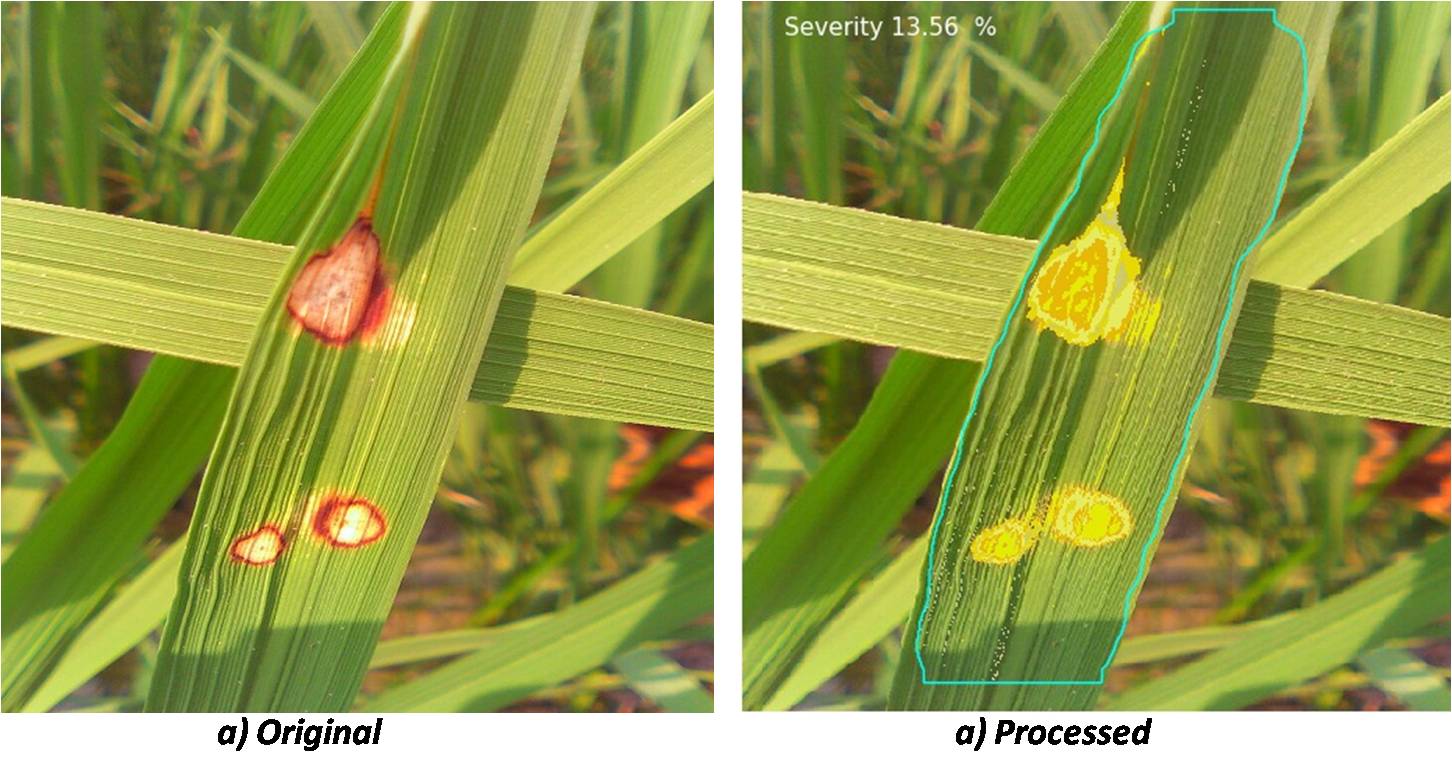
Sample image processed by Imago AI showing the proportion of a crop affected by disease
In terms of where they are now, the bootstrapping, nearly year-old startup is working off data from a number of trials with seed companies — including a recurring paying client they can name (DuPont Pioneer); and several paid trials with other seed firms they can’t (because they remain under NDA).
Trials have taken place in India and the U.S. so far, they tell TechCrunch.
“We don’t really need to pilot our tech everywhere. And these are global [seed] companies, present in 30, 40 countries,” adds Goyal, arguing their approach naturally scales. “They test our technology at a single country and then it’s very easy to implement it at other locations.”
Their imaging software does not depend on any proprietary camera hardware. Data can be captured with tablets or smartphones, or even from a camera on a drone or using satellite imagery, depending on the sought for application.
Although for measuring crop traits like length they do need some reference point to be associated with the image.
“That can be achieved by either fixing the distance of object from the camera or by placing a reference object in the image. We use both the methods, as per convenience of the user,” they note on that.
While some current phenotyping methods are very manual, there are also other image-processing applications in the market targeting the agriculture sector.
But Imago AI’s founders argue these rival software products are only partially automated — “so a lot of manual input is required,” whereas they couch their approach as fully automated, with just one initial manual step of selecting the crop to be quantified by their AI’s eye.
Another advantage they flag up versus other players is that their approach is entirely non-destructive. This means crop samples do not need to be plucked and taken away to be photographed in a lab, for example. Rather, pictures of crops can be snapped in situ in the field, with measurements and assessments still — they claim — accurately extracted by algorithms which intelligently filter out background noise.
“In the pilots that we have done with companies, they compared our results with the manual measuring results and we have achieved more than 99 percent accuracy,” is Goyal’s claim.
While, for quantifying disease spread, he points out it’s just not manually possible to make exact measurements. “In manual measurement, an expert is only able to provide a certain percentage range of disease severity for an image example; (25-40 percent) but using our software they can accurately pin point the exact percentage (e.g. 32.23 percent),” he adds.
They are also providing additional support for seed researchers — by offering a range of mathematical tools with their software to support analysis of the phenotypic data, with results that can be easily exported as an Excel file.
“Initially we also didn’t have this much knowledge about phenotyping, so we interviewed around 50 researchers from technical universities, from these seed input companies and interacted with farmers — then we understood what exactly is the pain-point and from there these use cases came up,” they add, noting that they used WhatsApp groups to gather intel from local farmers.
While seed companies are the initial target customers, they see applications for their visual approach for optimizing quality assessment in the food industry too — saying they are looking into using computer vision and hyper-spectral imaging data to do things like identify foreign material or adulteration in production line foodstuffs.
“Because in food companies a lot of food is wasted on their production lines,” explains Gupta. “So that is where we see our technology really helps — reducing that sort of wastage.”
“Basically any visual parameter which needs to be measured that can be done through our technology,” adds Goyal.
They plan to explore potential applications in the food industry over the next 12 months, while focusing on building out their trials and implementations with seed giants. Their target is to have between 40 to 50 companies using their AI system globally within a year’s time, they add.
While the business is revenue-generating now — and “fully self-enabled” as they put it — they are also looking to take in some strategic investment.
“Right now we are in touch with a few investors,” confirms Goyal. “We are looking for strategic investors who have access to agriculture industry or maybe food industry… but at present haven’t raised any amount.”
https://techcrunch.com/2018/11/29/agtech-startup-imago-ai-is-using-computer-vision-to-boost-crop-yields/